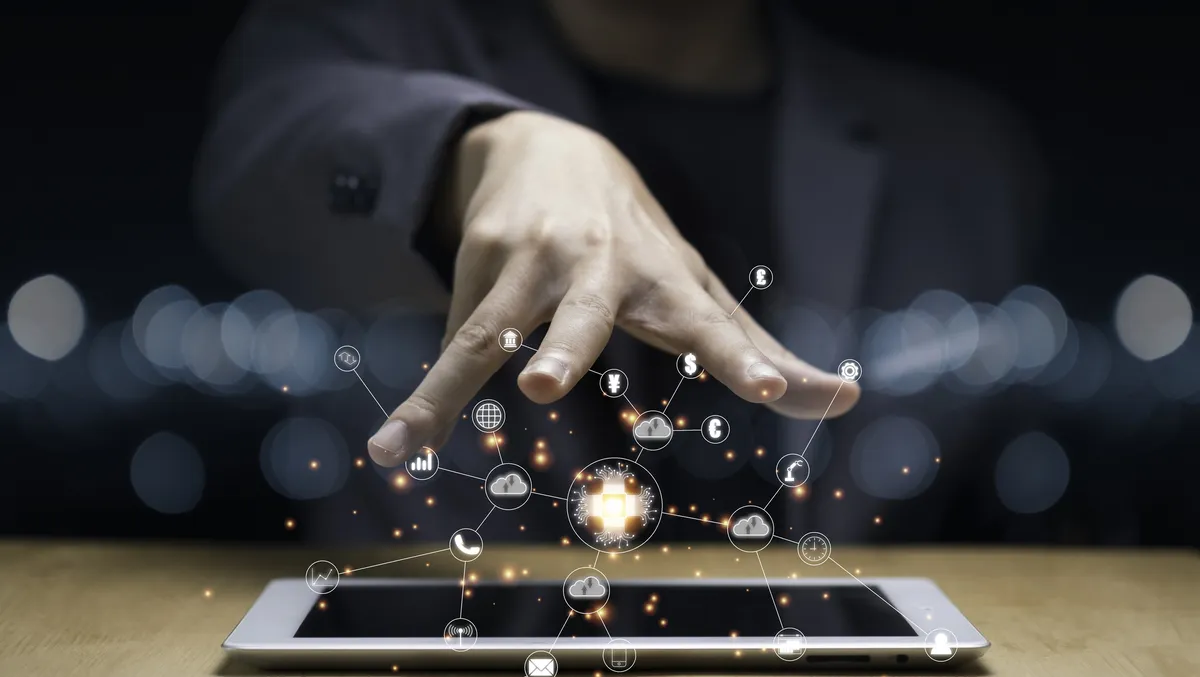
Alternative Data: Insights, Markets, and Decay of Value
Alternative data has shaken up the world of investing and business. Previously, informational sources were limited to official documents (e.g. company and government reports), media (e.g. newspapers), and internal company information. Now companies have access to information that has been produced independently.
According to our survey on the financial sector, alternative data has been deployed by over 60% of businesses. Based on a study done by Deloitte, the spending on big data analytics has overstepped $3 billion in the US.
Clearly, the fundamental idea of alternative data works. However, should such data sources be treated as any other that has previously existed? I believe that there are significant differences between alternative and traditional data. Namely, in the way they provide value.
What's in an insight?
A lot of people throw around magnificent words like "insights" and "value". In our case, these would be words we often come across in the field of data. Supposedly, someone wrestles insights and value out of a dataset, and the company then takes action.
There are many ways to go around defining the word "insight". However, taking the context into account, I'd say a fairly good definition would be that insights are highly likely predictions. These predictions can be about any part of the business, such as the historical performance of a particular department or person. They can equally be predictions about the future.
Some obvious conclusions can be derived from the definitions (e.g. that the accuracy of insights heavily positively correlates with the accuracy and quality of data). However, what I find of interest are actionable insights. In order words, insights that are supposed to be acted upon.
Of course, there's an implicit assumption that actionable insights have a positive expected value over an undefined period of time. In other words, acting upon them will produce more ROI over the long term rather than less. However, if we closely follow the reasoning so far, we can notice that while predictions are objective statements, actions are interpretations of predictions.
To simplify a little bit, let's take an example of a dataset that shows, with confidence, that a particular product of one company might experience a massive surge in demand. What actions would be taken by a hedge fund, and what actions would be taken by a competitor of the company in question? Clearly, the prediction is the same, but it means different things to different businesses.
Under the weight of markets
Most economists agree that competition, outside of fringe cases, is not only a healthy part of business but also an integral part of the entire economy. In short, as businesses compete for the limited spending power of the consumer, they have to find ways to optimise their operating model to outperform competitors.
Over all the business models in the economy, competition takes different forms and shapes. Some companies, such as SaaS, might choose to improve on their features, customer service, etc. Businesses that produce physical products might look for improvements in manufacturing and distribution processes.
As an example of optimisation through alternative data, we can pick Walmart. According to an Eagle Alpha report, Walmart has a Data Cafe that automatically pulls data from over 200 sources (including such sets as meteorological and social media data). All of that information is processed in mere seconds to produce actionable insights for the company.
However, my previously mentioned financial sector is in a unique position. If we were to simplify and idealise the industry by a wide margin, its main function is continually moving the capital to those who can maximise the further production of profit.
Companies in the financial sector do this by using any information possible to deduce who will be those maximising profit. Since there's no better way to get as close to accurate predictions other than crunching numbers, data is the answer to their woes. Of course, that is the reason why alternative data has taken hold so quickly and so powerfully.
Yet, there's an issue that creates competition. Companies can't maximise output for an infinite sum of capital. Or, more simply, a startup can effectively utilise some N amount of money, while an established enterprise might be able to effectively utilise significantly more (even then, the amount is not infinite).
Thus, there's a race to be the first.
Decay of value in alternative data
There's an old idea in finance that public information is essentially worthless. It's assumed that markets adjust to any new public information nearly instantaneously, making consistent alpha generation impossible.
However, let's go back to our previous example. Clearly, if an important product of a company is about to experience a massive surge in demand, it would make sense to buy some stock beforehand. Yet, something interesting happens here because alternative data is public data.
All the alternative data is essentially accessible to anyone. There might be financial or technological barriers, but by its nature, it is public information. However, it seems to function as private data while being public since the markets have not adjusted to the possible insights.
Flipping the script again, we should expect markets to adjust to the insights provided by alternative data over time (or never, if the insights are never discovered). They just adjust much more slowly than with public data.
We can see that happening in nearly real time. According to a Grennich Associates study, over 20% of investment firms reportedly generate 20% of their total alpha through alternative data. Data that is essentially public.
Additionally, since there's a finite amount of financial resources that each company can utilise effectively, we can derive that these adjustments will be incremental. That is, the first person to act upon the insights provided will get the most with those who come after experiencing diminishing returns.
In short, alternative data is a completely different type of information. Its value experiences slow erosion over time when markets start adjusting. However, they only start doing so once the first insight within a particular dataset is discovered and acted upon.
Conclusion
There's an important lesson to be learned - velocity of discovery is important in alternative data. The incremental adjustments in markets mean that beating everyone to the punch is more effective than ever before.
In practice, that means those who invest more into high-speed alternative data acquisition methods and time-efficient analysis techniques will beat everyone else.